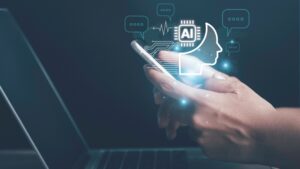
Monte Carlo in the present day rolled out a pair of AI brokers designed to assist knowledge engineers automate powerful knowledge observability issues, together with growing knowledge observability screens and drilling into the foundation trigger of information pipeline issues.
Monte Carlo has made a reputation for itself as one of many preeminent knowledge observability instrument suppliers. Whereas the corporate makes use of machine studying algorithms to detect knowledge pipeline anomalies, its choices have historically leaned closely on the experience of human knowledge engineers and knowledge stewards to grasp the context of information and knowledge relationships.
That’s beginning to change with the introduction of agentic AI capabilities into the Monte Carlo providing. At the moment, the corporate introduced two observability brokers, together with a Monitoring Agent and a Troubleshooting Agent, that it claims will dramatically pace up time-consuming duties that beforehand had been depending on human experience.
For instance, the brand new Monitoring Agent will enable clients to create knowledge observability screens with thresholds that make sense for the actual atmosphere that it’s being deployed in. That beforehand required the diligent work of an information engineer or knowledge steward to create thresholds that had been neither too noisy nor too permissive.
Discovering that Goldie Locks zone used to take people, however it may now be finished reliably with agentic AI, says Monte Carlo Area CTO Shane Murray.
“That normally requires plenty of enterprise context, requires plenty of understanding of the information and of the enterprise to have the ability to create these guidelines and to outline helpful alert thresholds,” Murray tells BigDATAwire. “What the monitoring agent does is it identifies subtle patterns throughout columns within the knowledge, throughout relationships, and basically profiles each the information to grasp the way it correlates and what are the potential anomalies that may happen within the knowledge; the metadata to grasp the context for the way it’s used; after which question logs to grasp the enterprise influence of these. After which it suggests to the person a sequence of suggestions.”
Monte Carlo had already began to dabble with agentic AI. In late 2024, it gave clients the power to have generative AI recommend monitoring guidelines, which is what grew to become the Monitoring Agent. The corporate has a number of clients already utilizing this providing, together with the Texas Rangers baseball staff and Roche the pharmaceutical firm. Collectively, these early adopters have used the GenAI to create hundreds of monitor suggestions, with a 60% acceptance price.
With the rollout of the Monitoring Agent, the corporate is taking the subsequent step and giving clients the choice of placing these observability screens into manufacturing, albeit in a read-only method (the corporate isn’t letting AI make any adjustments to the methods). In accordance Lior Gavish, the CTO and co-founder of Monte Carlo, the Monitoring Agent will increase monitoring deployment effectivity by 30 p.c or extra.
The Troubleshooting Agent, which is at the moment in alpha and at the moment scheduled to be launched by the tip of June, goes even additional in automating steps that beforehand had been finished by human engineers. Based on Murray, this new AI agent will spawn a number of sub-agents to fan out throughout a number of methods, reminiscent of Apache Airflow error logs or GitHub pull requests, to search for proof of the reason for the information pipeline error.
“What the troubleshooting agent does is it really assessments a lot of these hypotheses about what may have gone fallacious,” Murray says. “It assessments it within the supply knowledge. It assessments it throughout potential ETL system failures, varied code which have been checked in.”
There could possibly be tons of of subagents spawned that can all work in parallel to seek out proof and check speculation about the issue. They may then come again with a abstract of what they discovered, at which level it’s again within the arms of the engineer. Monte Carlo says early returns point out the Troubleshooting Agent may scale back the time it takes to resolve an incident by 80%.
“I see this as going from root trigger evaluation to being very guide and basically taking days or perhaps weeks right down to a state of us supplying you with the instruments so you could possibly doubtlessly do it in hours,” Murray says, including that it’s basically “supercharging the engineer.”
With each of those brokers, Monte Carlo is attempting to copy what human staff would do by analyzing knowledge after which taking acceptable subsequent steps. Monte Carlo is searching for extra AI brokers to construct to additional streamline knowledge observability for purchasers.
The 2 AI brokers are based mostly on Anthropic Claude 3.5 and run completely in Monte Carlo’s atmosphere. Prospects don’t have to arrange or run a big language mannequin or pay an LLM supplier to utilize them, Murray says.
Associated Gadgets:
Will GenAI Modernize Information Engineering?
Monte Carlo Brings GenAI to Information Observability
Monte Carlo Detects Information-Breaking Code Adjustments